Establishing Ethical AI Governance in Responsible & Trustworthy AI Development
- McCoyAle
- Feb 14
- 10 min read
Updated: Feb 19
Artificial Intelligence (AI) has become an integral part of modern society, influencing various sectors from healthcare to finance and fostering important dialogue related to its governance within the American government system. As the technical capabilities continue to advance, so does the challenges associated with ensuring its ethical use. With each innovative idea brings about a new capability to assess how we responsibly build AI centered software and technology. The need for robust AI governance frameworks has never been more critical than now. In this article we'll explore the current state of AI governance, examine available frameworks, algorithms, and tools that promote ethical and responsible AI systems.
Understanding AI Governance
AI governance refers to the frameworks, policies, and practices in place to guide the design, development, deployment, and use of AI technologies. It encompasses not only compliance with regulations but also ethical considerations that influence how AI impacts society. The rapid evolution of AI technologies poses unique challenges, including bias in algorithms, lack of transparency, and potential misuse. Therefore, effective governance is essential for building trust and ensuring that AI benefits everyone, while each solution is tailored to meet the requirements of the intended persona journey.

AI Governance Types
Within various industries, including the federal government, are AI advances prompting the concerns surrounding governance structures. There is a critical need for businesses and individuals to ensure ethical datasets and algorithms are used in AI which are fair to all users and represent diversity of intended audiences. The Responsible AI Institute, actively involved in the policies and procedures surrounding AI development, has outlined 5 data types through its initiatives developing ethical frameworks. Depicted in the image above you'll find references to examples of each AI governance type.
When considering the governance type(s), it's equally important to understand the "type" of law being covered as well as the approach that is required, especially when building and taking future scalability into consideration. According to Responsible AI Insititute's (RAI) framework, you'll want to:
Differentiate between Hard Law and Soft Law
Hard laws are laws established by a governing body, and are likely to have levels of repercussions to them. While, soft laws act as guidelines for how AI systems should be created and managed to mitigate certain risks and outcomes.
Differentiate between Horizontal and Vertical Approaches
An horizontal approach will focus on guidelines and policies that act as industry standards or span an entire domain, while a vertical approach will focus on a single domain or sector within a domain. In respect to AI systems, the policies and guidelines being developed by global organizations and governments span the AI space across industries. However, within AI, you may encounter different policies and procedures establish for healthcare systems, than those supporting financial or security systems. To add another layer, within each area storage is an important aspect of maintaining critical data while networking plays a critical role to how data is transferred. Each within separate industries will still maintain foundational procedures for how things need to be implemented and managed despite the industry, although configuration options and certain settings are specific to the associated use case.
The Importance of Ethical AI
Ethical AI prioritizes fairness, accountability, and transparency in AI systems. This means that developers need to actively consider and mitigate any potential bias in their algorithms. The implications of unethical AI practices can be severe, leading to discrimination and loss of public trust. In some instances, these can result in legal fines and monetary compensations to relevant entities.
To ensure ethical AI development, organizations must implement governance frameworks that promote these values from the ideation stages of the AI lifecycle. The societal impact of AI requires a collaborative approach, where stakeholders also include developers, policymakers, and the relevant communities—working together to establish standardized guiding principles.
Trustworthy AI Concepts
According to NIST, there are 7 concepts that need to be taken into consideration when evaluating your AI system for Trustworthiness, setting the stage for building ethical systems.
Accountable and Transparent
Accountability within an AI system relates to its internal ability to address harmful or unfair outputs. Each output of an AI system must align with the decision frameworks built into the underlying system. While being transparent equates to making the decision frameworks and algorithms used available to consumers, ensuring the appropriate and necessary disclosures are available.
Valid and Reliable
Validation ensures the AI system is able to continuously output valid outputs that aligns with the originating use case, while maintaining its ability to be reliable, accurate, and robust. Robustness focusing on its ability to perform reliably under various conditions, over time, while outputting the best and most accurate decisions.
Safe
Safety involves an AI system's ability to output decisions that align with the moral and value system of humans. It's important to not limit these concepts to the harmful biases that are represented through AI bots and Generative AI platforms. This includes the decisions made by AI systems which focus on decision making in biotechnology, autonomous driving, AI robotics and various other industries and sectors.
Secure and Resilient
In a digitized society where new methods for cyberattacks occurs within various geographies its even more critical to ensure secure and resilient systems. This includes new considerations for protecting the Intellectual Property(IP) of AI users creating developments with AI systems. This is in addition to protecting relevant data, securing access and technical protects related to APIs and backend infrastructure.
Explainable and Interpretable
With rapid advances comes the ability of the AI organization to communicate and present the decision making process to consumers. This is especially true when communicating with end-users opposed to technical communities. Ensuring the appropriate disclosures and necessary information is availble to not only protect, but advocate for the validity of your systems is key to its success.
Privacy-Enhanced
Incorporating privacy enhanced concepts into AI systems to protect the identification of users and minimize privacy risks. Although important to all AI systems, it's critical to areas within government sectors and cybersecurity and intelligence domains.
Fair with Harmful Bias Managed
When making an AI system available to consumers, ensuring the eliminations of unfair or biased decisions is critical. Utilizing frameworks and systems to identify gaps and blind spots where unintended bias can occur, is important to not only the success of the AI system but the longer term scalability and innovation that can happen when chances of bias and anomolies are not detected. The development of bias detection software is still a growing area.
Key Frameworks for AI Governance
Several frameworks have been developed to provide guidance on the ethical use of AI technologies. Frameworks act as guidelines for policies and procedures needed to think through the relevant areas and concepts that will help set your AI system up for future success. Some of the most prominent include:
The EU's Ethics Guidelines for Trustworthy AI
The European Union has published guidelines that outline key requirements within 7 areas for an AI system to be considered as trustworthy. These guidelines emphasize the need for AI systems to maintain human agency and oversight. They also include an AI systems ability to be private, robust, transparent, diverse, and accountable, similar to the referenced list in an earlier section.
One differentiator includes the explicit mention of environment safety, eliminating the ability to include it as a humane safety concern or bias. Each area focuses on key principles to remain accountable, and non-discriminatory.
The OECD Principles on Artificial Intelligence
The Organisation for Economic Co-operation and Development (OECD) provides a set of principles aimed at promoting the responsible development of AI. These principles encourage governments and stakeholders to ensure AI systems respect human rights and democratic values while being designed to promote inclusive growth and countries are able to adhere to the set of principles.
The IEEE Global Initiative on Ethics of Autonomous and Intelligent Systems
The IEEE-focused initiative advocates for ethical considerations in the development of autonomous systems. It promotes frameworks that align technology with human well-being, proposing design processes that include ethical impact assessments. A critical aspect to ethical development of intelligent systems is the ability to eliminate misunderstandings and misappropriations that are faced when developing sociotechnical AI systems.
As a reference, sociotechnical systems incorporate the technical and society (or community) aspects, independently but as subsystems within a larger system.
Algorithms and Tools for Ethical AI Development
In addition to governance frameworks, various algorithms and tools can enhance ethical AI practices. Before centering the discussion around each, let's take a moment to understand the AI Lifecycle for developing Ethical AI Solutions.
Plan and Design
Developing the responsible, legal and technical scope of the AI project to establish the governance principles in preparation for design and build steps.
Collect and Process Data
Collecting, aggregating, and assessing data sources and relevant data to ensure diverse representation of target audience. It's important to understand diverse representation in the scope of the variation(s) in data being used.
Build and Use Model
Desinging, developing or fine-tuning specific Large Language Models (LLMs) that are used to achieve the objectives to solve the broader problem statement established. In this stage, it is critical to monitor and assess the performance of the model, as it relates to the guiding principles.
Verify and Validate
Verification and Validating the system consists of the testing that the solution meets the intended goals and align with the initial specifications. Its important to understand the verification and validate phase differs from the build and use model, in that, the former focuses on the algorithms built into the model while validation refers to the usability of the solution related to the intended use case and audience.
Deploy and Use
Deploying an AI system consists of a similar series of steps that are used in an environment for software and technology solutions. This includes ensuring available hardware is available, all system aspects have been configured and operational use is available for the intended consumers and relevant stakeholders.
Operate and Monitor
Similar to other technical systems, the need for ongoing assessments, relevant modifications and patches, and updates relevant to software, hardware, and policy are required to ensure the integrity of the AI system.
Although the steps required within each phase are similar to the development of many software and technology processes, its important to understand the relevant data and artifacts associated with each step to ensure the integrity and data freshness throughout its lifecycle.
Although not explicitly mentioned, the incorporation of a sunset or decomission phase within the lifecycle, and specific to AI systems, should be taken into consideration for recommendations related to artifacts or hardware that might require different handling from a normal technology system. Similar, to the lifecycle depicted in the following image, and listed in OECD's lifecycle.

Let's flow through a few algorithms and tools that will help ensure steps within each of the referenced phases are completed with the necessary diligence.
Fairness-Aware Algorithms
These algorithms are designed to identify and reduce bias in AI systems. They typically consist of a three step process of pre-processing data, in-processing, and then post-processing. For instance, techniques such as adversarial debiasing and re-weighting training data help ensure that AI models perform equitably across different demographic groups by training models use biases to detect bias.
Explainable AI (XAI)
One of the major challenges with AI systems is the "black box" issue, where the reasoning behind an AI's decision-making is unclear. XAI is a separate field of study which focuses on helping consumers understand AI's decision making process and how to improve and evaluate models and their prediciations. It is one of the concepts that largely contributes to enhancing transparency and trust, contributing to building more ethical AI systems.
AI Audit Tools
AI Auditing Tools consists of tools that are used to assess whether an AI system complies with the necessary ethical guidelines. These tools identify trends and patterns in data where bias and other non-compliant behaviors occurs. Auditing tools provide similar benefits to that of automation, improving efficiency through the elimination of human error by maintaining control of the auditing lifecycle. Currently the Instititute of Internal Auditors (IIA) uses the Artificial Intelligence Auditing Framework to help organizations reduce risks by implementing a set of best practices when implementing AI Systems.
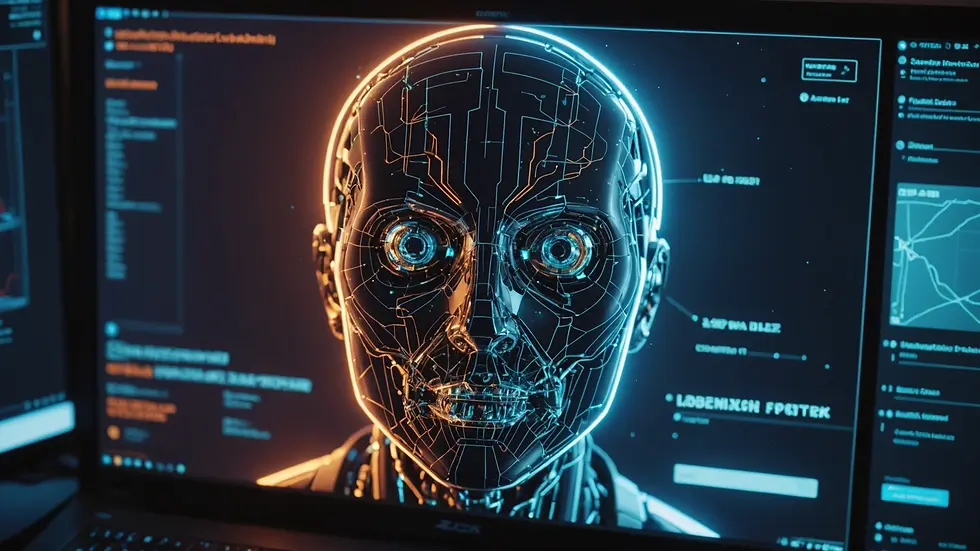
The Role of Stakeholders in AI Governance
The standardization of ethical AI is not solely the responsibility of AI developers. Stakeholders, including government entities, industry leaders, and civil organizations, must collaborate to create an inclusive dialogue about AI governance. This includes contributing to and adopting relevant governing frameworks to implement and contribute feed findings back towards.
Government Entities
Regulatory bodies play a crucial role in establishing rules and standards for AI use. Increased collaboration between federal regulators, technology executives, analysts and scientists is continously occurring. Governments are tasked with creating legislative frameworks that promote ethical AI while still balancing innovation and public safety.
AI Developers and Organizations
AI developers must integrate ethical considerations into their design processes. This involves continuous education on the implications of AI technologies and the adoption of ethical practices throughout the project lifecycle and feeding back relevant findings to continue the advancement of these design processes and framework phases.
Civil Society
Civil society organizations, including advocacy groups, consulting firms, and non-profits, serve as major contributors and overseers by holding both institutions and companies accountable for relevant AI practices, ensuring they are put to good use. Their input is essential for keeping up with and understanding societal impacts, while ensuring a diverse range of perspectives is represented in the governance dialogue.
Challenges in AI Governance
Despite the frameworks and tools available, several challenges still remain in the AI governance pipeline, and should be taken into consideration as early in the AI system's lifecycle as possible. A few of these challenges include:
Rapid Technological Advancements
AI technology continues to evolve at an unprecedented pace, outpacing the development of regulatory frameworks and creating innovative startups tackling key problems within specific industries and sectors. This creates a gap where ethical concerns may not be addressed in real-time, but can be improved through utilizing and contributing to a governing body when necessary and feasible.
Lack of Standardization
The rapid growing of standardized regulations across countries complicates global AI governance efforts, leaving organizations to adopt pieces of governance structures that are relevant to a specific use case. Without common legal and ethical standards, ensuring standardized ethical practices can be challenging, especially for multinational organizations. It's important to include standards that are relevant to your country, and also seek out governing standards that your country participates in.
Resource Limitations
Smaller organizations may face difficulties in adopting a more comprehensive governance framework or implementing various tools to support guidelines within frameworks and toolkits, due to resource constraints. As such, equitable access to advice and tools, publicly available is essential in creating a more uniform approach to ethical AI development.
Final Thoughts
As AI continues to shape the future of society, the importance of implementing effective AI governance becomes more critical. AI governance incorporates critical guidelines related to data science, engineering, policy and security, of which require input from various entities and subject matter experts to validate its unified and safe usage. Understanding and utilizing available frameworks, algorithms, and tools, enables stakeholders to develop ethical and responsible AI systems with confidence and guardrails. Collaboration among governments, engineers, and civil society is key to creating an inclusive approach that guarantees societal trust and ethically maximizes the positive impact of AI technologies.
Moving forward, it is important for all relevant stakeholders to remain vigilant and committed to fostering ethical AI development that prioritizes the well-being of society. In a digital and consistently shifting technological world, proactive safe governance will be the cornerstone of ethical AI development and rapid adoption and usage among everyday consumers.
Comments