Mastering the Basics of Data Analytics: A Complete Guide to Getting Started
- McCoyAle
- Feb 4
- 6 min read
Technology is consistently advancing, while improving practices such as Reliability Engineering. In a previous article, "Reliability Engineering: Service Level Quantifiers," we discussed the principles of Reliability Engineering with service level metrics used to measure system performance. These metrics are used to build Service Level Indicators (SLIs), which are monitored by Service Level Objectives (SLOs) or target thresholds. The goal of both is to measure system performance as it relates to the customer experience vs. resource consumption and technical issues. To establish and maintain each requires effective implementation of observability, monitoring, and alerting frameworks and tooling. Driving each of these aspects is the underlying data.
Data, related to systems and the customer experience, has become an essential skill in today’s data-driven world. This makes it imperative for organizations to understand how to collect, aggregate, and examine the appropriate data to draw accurate conclusions related to relevant information. As organizations strive to make more informed decisions that impact the broader business, the importance of effectively interpreting data and the implications of the data's impact on the business becomes more critical. Whether you're looking to enhance your ability to make data driven decisions related to your business or simply want to understand the power of data analytics, mastering the basics is the first step to getting started.
In this article, we'll cover the fundamental concepts of data analytics, the tools you can use, and practical steps to get you started on your journey.
Understanding Data Analytics
Data analytics refers to the comprehensive process of examining, cleaning, transforming, and modeling data sets with the ultimate goal of drawing insightful conclusions about the information they contain. In some instances, such as data analysis and reporting or machine learning, you might hear the term "ETL" referenced. This is related to extracting, transforming and loading data from difference sources into a single database or data-warehouse.
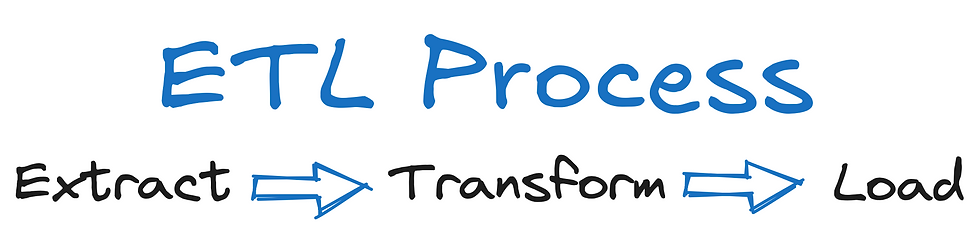
This delicate practice consists various stages, techniques, and tools that are designed to convert raw data into meaningful insights that improve the capabilities of processes for informed decision-making. It is often thought that he journey of data analytics begins with data collection, where vast amounts of information are gathered from various sources. It begins with the discovery phase to effectively define and create a problem statement. Before focusing on the characteristics of data, it's important to first understand the Data Analytics Lifecycle.

Data can be categorized into two primary types:
Structured Data - Is data that is organized in a defined manner, such as in relational databases or spreadsheets.
Unstructured Data - Is data that lacks a pre-defined format, such as text from social media posts, images, and videos.
The process and steps within each phase can vary significantly in complexity, ranging from simple descriptive statistics, which summarize past data through measures such as mean, median, and mode, to more sophisticated techniques like inferential statistics and complex predictive modeling that utilize algorithms to forecast future trends based on historical data patterns. Much of these variations are used within Reliability Engineering and Observability to build metric based queries for advanced decision making capabilities.
Mastering the Art and Science of data analytics helps to gain the invaluable ability to make informed decisions grounded in solid data evidence rather than relying solely on intuition or gut feelings. Embedding data analysis into internal capabilities will positively influence business strategies and outcomes, while also enabling organizations in other areas to better identify market trends, optimize operations, and ultimately drive profitability. In a society where data is often referred to as the new oil, the capacity to analyze and interpret data effectively is becoming increasingly critical for driving success across various industries.
Types of Data Analytics
Understanding the different types of data analytics is essential for anyone starting in this field, as it ensures the appropriate methods and techniques are used for managing and analyzing data. More importantly, it will play a critical role in how data is presented to create the correct narrative for the targeted audience. Four of the main types of data typically handled are:
Descriptive Analytics
Descriptive analytics focuses on summarizing historical data to understand what has happened in the past. It provides insights through measures such as averages, totals, and percentages.
For example, a retail company may analyze historical sales data to determine peak shopping times or seasonal trends.
Diagnostic Analytics
This type investigates why something happened by interpreting historical data. It often includes more complex analytical methods, such as identifying correlations between different variables.
For instance, if sales dropped during a particular period, diagnostic analytics would help find the causes—whether it was lower customer traffic or increased competition.
Predictive Analytics
Predictive analytics uses statistical models and machine learning techniques to forecast future outcomes based on historical data. This approach is useful for anticipating future trends and behaviors.
For example, a company might use predictive analytics to determine potential sales growth by analyzing current market trends and historical performance data.
Prescriptive Analytics
Prescriptive analytics builds on predictive analytics by recommending actions you can take to achieve desired outcomes. This method often utilizes optimization algorithms and simulations to determine the best course of action.
For example, a transportation company could use prescriptive analytics to optimize delivery routes and minimize shipping costs.
Essential Tools for Data Analytics
To embark on your journey into data analytics, familiarizing yourself with key tools is crucial. Here are some popular tools used in the data analytics field:
Microsoft Excel
Excel is a powerful tool for basic data manipulation and analysis. With features like pivot tables, chart creation, and various functions, it serves as an excellent starting point for any aspiring data analyst.
SQL (Structured Query Language)
SQL is essential for querying databases and manipulating structured data. It allows analysts to retrieve and analyze data efficiently. As data is often stored in databases, proficiency in SQL is a valuable skill.
R and Python
Both R and Python are programming languages widely used in data analytics. R is particularly strong in statistical analysis, while Python offers versatility and a wealth of libraries for data manipulation and visualization.
Familiarity with either of these languages will enhance your analytical capabilities significantly.
Tableau
Tableau is a leading data visualization tool that enables users to create interactive and shareable dashboards. With its user-friendly interface, individuals can turn complex data into easy-to-understand visual insights.
Steps to Mastering Data Analytics
Now that you have a basic understanding of data analytics and its tools, here are steps you can take to enhance your skills further:
1. Start with Data Fundamentals
Before diving into analytics techniques, it's important to have a solid understanding of data fundamentals. This includes knowing about different data types, data structures, and common storage methods.
Understanding these concepts will provide a strong foundation for your analytical journey.
2. Get Hands-On Experience
Theory is important, but practical experience is crucial in data analytics. Begin with small datasets to practice your analysis. Use Excel or programming languages like R or Python to manipulate data and generate insights.
Hands-on experience will enhance your learning process significantly.
3. Learn Analytical Techniques
Familiarize yourself with key analytical techniques, such as regression analysis, hypothesis testing, and data cleaning methods. Online courses, tutorials, or even textbooks can offer structured learning paths.
Continued practice with these techniques will help reinforce your understanding.
4. Work on Real-World Projects
Apply your analytic skills to real-world projects. This could involve volunteering for non-profits, participating in internships, or working on personal projects that interest you.
Real-world applications will enhance your portfolio and improve your problem-solving abilities.
5. Stay Updated with Industry Trends
The field of data analytics is continuously evolving, with new tools and techniques emerging regularly. Subscribe to relevant blogs, attend webinars, or join online communities to stay updated on industry trends and best practices.
Networking with other data professionals will also provide additional learning opportunities.
Conclusion
Understanding the basics of data analytics is an invaluable skill in today’s job market. It is one that can significantly enhance both career prospects and personal development. In an society where data is often overlooked, possessing a solid foundation in various types of analytics, descriptive, diagnostic, predictive, and prescriptive will improve the ability to effectively interpret and utilize data to make informed decisions. Understanding the characteristics of analytics serves a unique purpose in scenarios for understanding historical data trends and forecasting future outcomes based on current and past data. This enables businesses to not only analyze data but also to communicate insights effectively to stakeholders.
Familiarity with the underlying characteristics ensures the right analytical tools and techniques are selected for the job. Simple but effective tools like Excel to more robust softwares implementing SQL, Python, and R capabilities, in tandem with data visualization platforms like Tableau and Power BI, are essential in the toolkit of a data analyst. Creating compelling visual representations of data with effective story telling strategies can significantly enhance one’s ability to convey complex information in a digestible format.
From healthcare to finance, marketing to operations, the ability to analyze and interpret data is a downstream dependency of effective decision making. Organizations are constantly looking for individuals who can provide insights that lead to strategic improvements and competitive advantages in their respective sectors. It is important to remember that continuous learning and practical application are key to becoming proficient in this growing domain. Investing time and effort into mastering the basics of data analytics not only equips you with essential skills but also prepares you for a future where data-driven decision-making is paramount and keeping pace with Artificial Intellingence (AI) and Machine Learning (ML) advances.
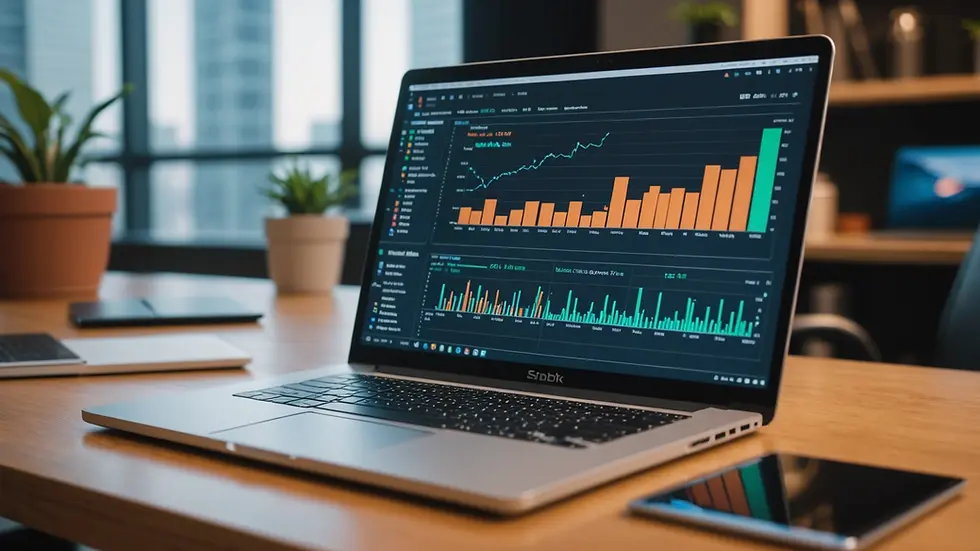
By following this complete guide, you can pave the way to becoming a proficient data analyst and unlock the full potential that data has to offer. Happy analyzing!
Comments